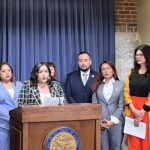
Panelists discuss potential of AI in healthcare
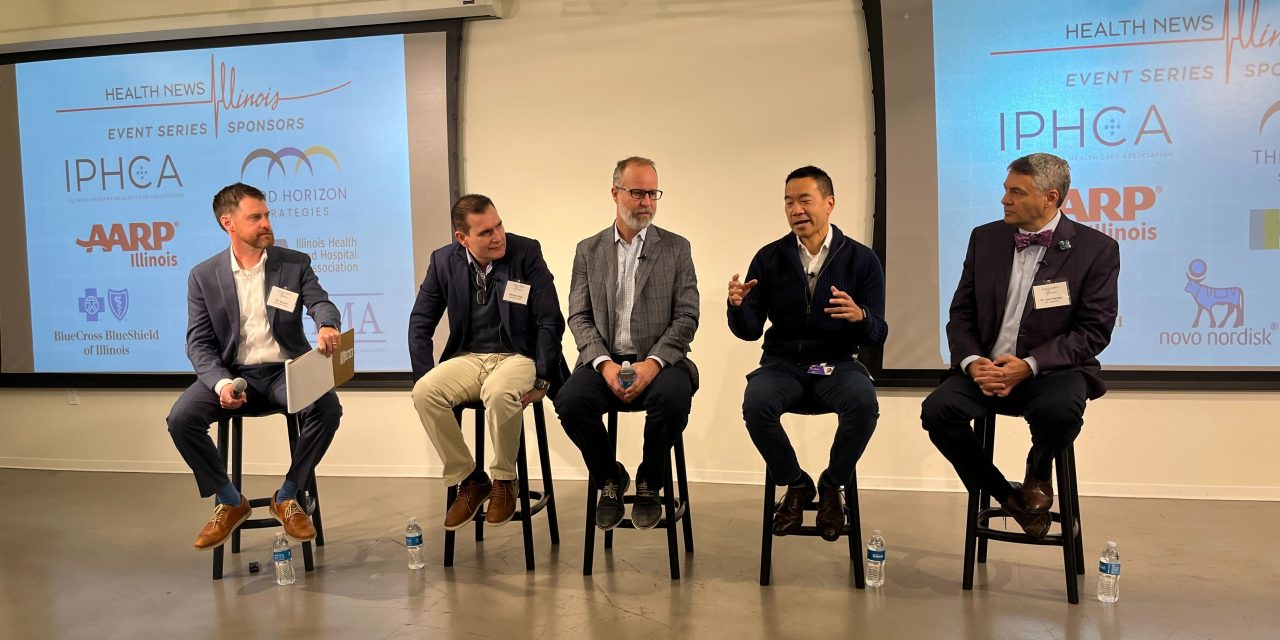
Experts at a Health News Illinois event Thursday said artificial intelligence is set to transform healthcare delivery, although unknowns remain on how the technology will advance in the coming years.
Speakers also weighed in on how AI may help the healthcare workforce and how to build trust in it among physicians and patients.
Panelists were:
- Dr. Jon Handler, Senior Fellow, Innovation, OSF HealthCare
- Chad Konchak, Assistant Vice President of Clinical Analytics, NorthShore University HealthSystem
- Dr. Abel Kho, Director, Institute for AI in Medicine, Northwestern University Feinberg School of Medicine
- Farrukh Khan, Founder & CEO, Inference Analytics, Inc.
Edited excerpts are below.
HNI: Where are things now with AI in healthcare?
Dr. Abel Kho: When this really broke out on the scene a year ago, for those of us who’ve been in the field, there were things that we could do that we never thought were possible. It’s not going to take us over, but it’s certainly going to help us quite a lot. I never thought I’d see the day when I didn’t have to write notes. That day has come, and I can’t tell you how excited I am about that one thing. So right now, in our case, we’re running a pilot to be able to see your patient and talk to them and have your phone there and recording. Then when you come out, you’ve got a note that’s probably better than I would have written myself. It catches a lot of things that you forget. Right now, at this point, we’re in the honeymoon phase. We’re all equally enamored with what’s possible, and we are seeing those early possibilities.
Dr. John Handler: We use artificial intelligence for a whole host of different uses in the healthcare system. We use it to identify who’s at high risk of decompensating unexpectedly, so we can jump on patients who are going to get worse, hopefully before they actually get there and save lives or get them to a higher level of care. We can help take care of them. We look to identify who’s at high risk for readmission so we can put actions in place to make it less likely that we will either discharge them earlier than they need to be or to help keep them out of the hospital and keep them healthy. We have mortality predictors that help us to identify who are patients whom we should probably have a conversation with sooner rather than later, or at least to help prioritize a conversation about what they want for their end-of-life care. Throughout the continuum of what we do, we have artificial intelligence sprinkled within.
Chad Konchak: There’s a lot of buzz about it, but a lot of this is new branding for things we’ve been doing for quite some time. We’ve been doing predictive analytics, that’s been one part of the umbrella for a while. The tagline is that AI will not replace physicians — but physicians who use AI will replace physicians who are not using AI.
That’s generally been the theme of technology for a long time, right? This really helps accelerate identification of patients who are at the highest risk of a potential outcome. That’s predictive analytics. We can prioritize them for better care, extract more value from all of the data that we might have and summarize through natural language processing. So those are some of the things that we’re doing right now.
Dr. Kho alluded to the more sexy aspects of what we’re hearing now with large language models and generative AI. It’s not going to override the gestalt of the physician, which I think should reign supreme. But it could remove a lot of those administrative burdens that they have on the teams. If you think about that as an automation component of it, those are the things that we’re starting to look into on that edge, but it’s still really, really new.
HNI: What’s the future for AI? Is it going to live up to its hype?
Farrukh Khan: Back in 2018, when we didn’t have the luxury of having (large language models). You had to teach AI models English first. You literally had to tell them how English words were structured, what a sentence is, how words sequence each other. And you took that and you layered the medical context on top. Therefore, when we had our first models, every other prediction was a hallucination.
The advantage now is that you don’t have to start with the first principles of teaching a model English. You have open source frameworks for these language models that are available to you. You don’t have to use ChatGPT if you are worried about data security.
You have those frameworks, and now you build the knowledge on top. The excitement here is because image AI, which has been happening for a much longer time — in 2018, they had something called ImageNet, which was basically the idea of understanding how images are structured — has gotten to a point where it is much more sophisticated now.
Text had its moment, and it had its moment in the last couple of years, where now suddenly there is an overabundance of (large language models), and you can pick and choose what is the most appropriate one to solve your use case. There’s still skill involved in understanding how to tune it, how to use it and how to make sure that it doesn’t forget what it already knows. So there is complexity. But the point is, because now you have this ability, things become much faster.
Chad Konchak: The hype component of AI right now is just that everything’s getting lumped in. Like, how many times have you guys seen a vendor like, ‘Oh, we’ve got this AI.’ And it’s just like this logistic regression model that we’ve been doing for a really long time. That’s the problem right now with the dialogue, everything’s just getting the label of AI.
What we’re talking about here is, if we look back on a 100-year timeline, one of the four transformational changes in technology. It’s for two reasons. One is the interface and how we interact with machines. You used to have to type into a computer, or in the old days with the cards, and write specific instructions and tell the machine what to do, really specifically, in a way that it understood, and then it would execute those tasks. But that is completely changing because we can speak to machines just as we speak to our spouses, our friends or our colleagues at work. That’s the first fundamental change.
The other is the cognitive component. It’s the brain that the machine has, just like the brains that we have with a neural network to translate all of that and almost communicate back to us. Now it’s kind of like talking to a 3-year-old because the English that it understands is pretty immature, and it just makes stuff up. But that’s going to get better.
HNI: What will be AI’s biggest impact on healthcare workforce challenges?
Dr. John Handler: There are only so many doctors and caregivers and clinicians in the world. It’s hard and expensive to train them. It’s hard and it’s expensive to have them. We have a crisis in healthcare where we have an aging population, a population that is increasingly getting ill. We have an increasing shortage of doctors and nurses and clinicians, and there’s no way we’re going to be able to train them. There is no amount of efficiency improvement that I think is going to close that gap. The studies say that primary care physicians already should be spending 26 hours a day to handle a normal panel of patients. You could make them 100 percent more efficient and you’re not going to close the gap all the way.
The bottom line is that the world is changing a lot, and we’re going to see a change in all the ways that we spoke about. It’s going to increase our efficiencies. It’s going to hopefully increase our ability to create access for patients. It’s going to increase our ability to create quality care. And hopefully, this is where we have to think out of the box: What about all the people who have no access to clinicians? It’s easy to talk about the people who have access to clinicians already and making that better. What about the people that really just can’t access one? You’re 100 miles away from the nearest doctor or you can’t afford it. For those people, we’ve got to give them an option and — for the people like us who also maybe want additional options even if we have access to care — maybe this is a way to help close that gap.
HNI: What can be done to earn the trust of patients and providers?
Dr. Abel Kho: We had a big event at Northwestern and like 1,000 people showed up. We surveyed the whole audience (about AI). Medical students and residents, 90 something percent thought this was great. And then all the way down is like the guy who’s been around for 40 years. That guy was not really enthused about this.
We took that to mean that, like all technologies, adoption typically is asymmetric. But what that means to us is that we have to really double down and invest in our future. That means that for anyone who’s coming up through the Feinberg School of Medicine, we actually have created one of the first schools to have a curriculum that’s both on digital health and data science. AI is part of that. We’re creating as many opportunities as possible. But the flip side is also true. Not only are we educating the students, but the students themselves are becoming part of the educational process.
Dr. John Handler: Part of the problem is that AI is a big heterogeneous thing. Every model that everybody makes is not equal to every other model that everybody else makes. It’s like saying, ‘I don’t trust medical research’ because some medical research is not so good. Some is really, really good and it’s transformed the care that we do. And so part of the challenge is saying, ‘Is there any AI that’s any good?’ And it would be hard to imagine that if you asked the question that way, you wouldn’t get more people saying, ‘OK, I agree that maybe there’s some that is decent.’
I also think this brings up an important point. We may have to think very differently about validation. At the end of the day, validation is the engine that drives healthcare. What makes a doctor say they’re going to do something? You have to show them some data that it works. What makes an insurance company say that they’re going to pay for something? You have to show them some data that this works. How are you going to get the (Food and Drug Administration) to approve something? It’s all about data.